BCG has published a report that offers a simplified set of routes for financial institutions to navigate the recent 2023 trends of Artificial Intelligence and Generative AI (Gen AI).
To what can easily start as complex strategies for financial businesses to implement, this report synthesizes an approach towards a comprehensive process to a Generative AI roadmap that focuses on roles, skill-sets, and strategies for teams, design leaders and executives (within Financial Services), to successfully identify the transformative steps and necessary actions for making AI decisions in 2024+.
In summary, the report highlights:
- A forward-looking approach to Artificial Intelligence and Gen AI and the importance of a vision necessary to align correct cultures and people strategies.
- Identifies challenges with iterative approaches or tentative tweaks that try to unlock value.
- How competitive advantage in Gen AI relies on true commitments to change and a path beyond the previous goals and horizons.
- Integration into existing networks is key, but re-imagining traditional operations for Gen AI requires a complete transformation.
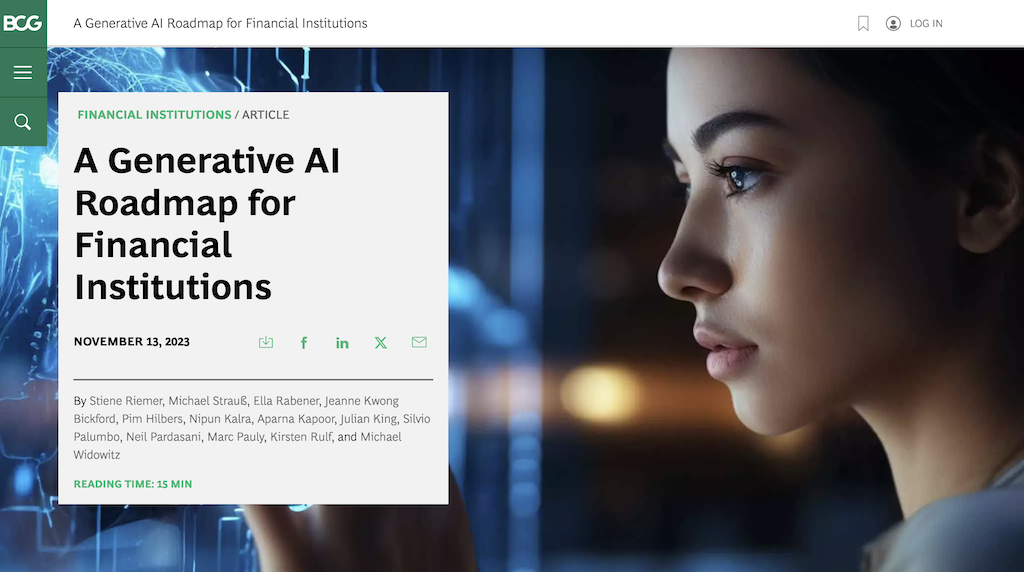
A shift is the extraction of value from existing AI processes, which tends to be based around predictive AI models that focus on:
- Behaviors
- Preferences
- Needs
- Risks
To new approaches which Gen AI introduces, which requires value extraction that AI models now focus on:
- Understanding
- Convergence
- Synthesis and
- Creation
It’s worth a read.
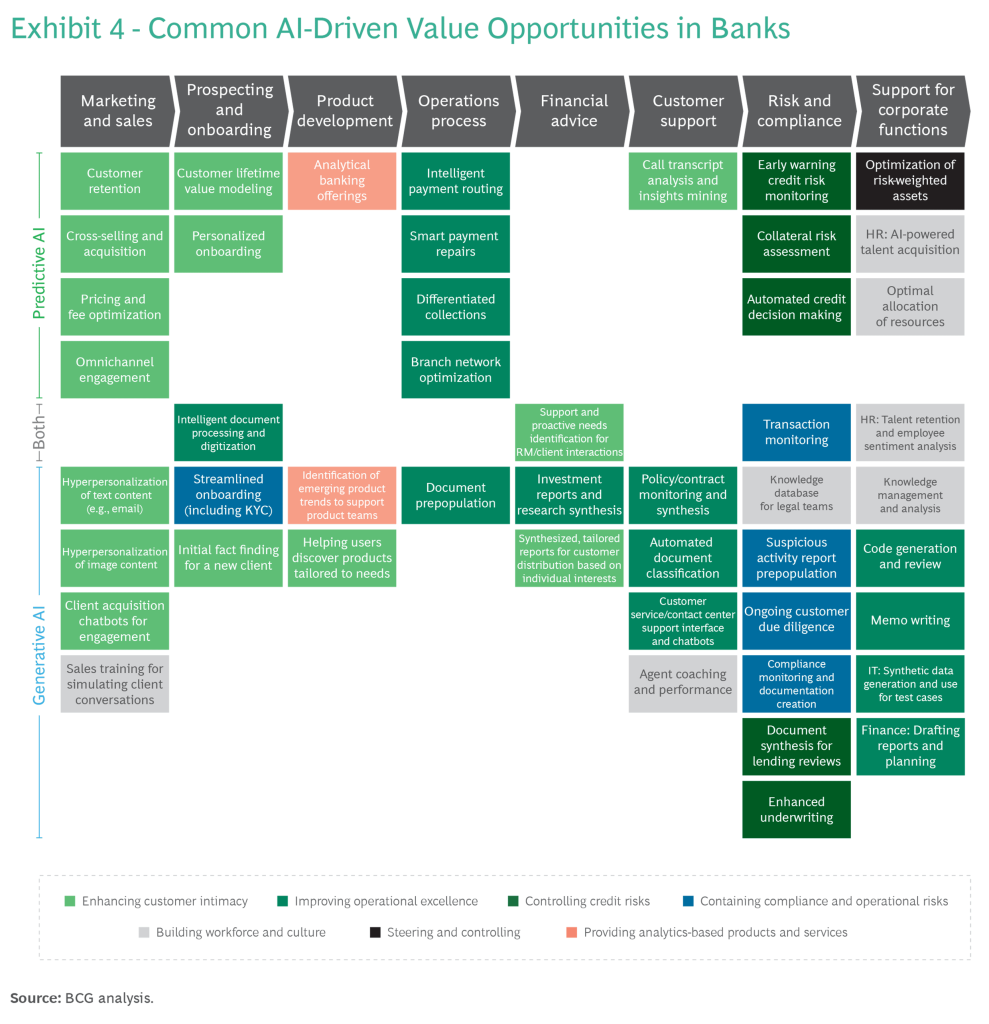
Source: BCG article – A Generative AI Roadmap for Financial Institutions (Nov 2023) – by Stiene Riemer, Michael Strauß, Ella Rabener, Jeanne Kwong Bickford, Pim Hilbers, Nipun Kalra, Aparna Kapoor, Julian King, Silvio Palumbo, Neil Pardasani, Marc Pauly, Kirsten Rulf, and Michael Widowitz
References (updated):
- Generative AI and the future of problem-solving – How thinking machines will change the way innovators work (December 2023) – Jonathan Kahan via Medium
- Navigating the Jagged Technological Frontier: Field Experimental Evidence of the Effects of AI on Knowledge Worker Productivity and Quality (September 2023) – Fabrizio dell’Acqua and Ethan Mollick in partnership with BCG
Some useful definitions:
- Artificial Intelligence (Classic Definition):
Refers to the simulation of human intelligence in machines that is programmed to think and learn like humans. It encompasses various technologies and techniques that enable machines to analyze, understand, and respond in a way that traditionally required human intelligence. - John McCarthy’s AI Definition:
Coined by computer scientist John McCarthy in 1956, Artificial Intelligence is the science and engineering of making intelligent machines, especially intelligent computer programs. It involves creating algorithms and systems that can perform tasks that typically require human intelligence, such as problem-solving, speech recognition, planning, and learning. - Modern Perspective:
In the contemporary context, Artificial Intelligence involves the development of algorithms and models that enable machines to process vast amounts of data, recognize patterns, and make decisions without explicit programming. Machine Learning and Deep Learning are integral components of modern AI, allowing systems to improve their performance through experience. - Narrow AI (Weak AI) vs. General AI (Strong AI):
Narrow AI refers to AI systems designed and trained for a specific task, while General AI aims to create machines with the ability to perform any intellectual task that a human being can. Currently, most AI applications fall under the category of Narrow AI, focusing on specialized functions like image recognition, natural language processing, and game playing. - Machine Learning:
A subset of AI, Machine Learning involves the development of algorithms that allow computers to learn from data. Instead of being explicitly programmed, these algorithms use statistical patterns to improve their performance over time as they are exposed to more data. - Deep Learning:
Deep Learning is a type of Machine Learning that involves neural networks with multiple layers (deep neural networks). Inspired by the structure and function of the human brain, deep learning has proven to be highly effective in tasks such as image and speech recognition. - Natural Language Processing (NLP):
NLP is a branch of AI that focuses on the interaction between computers and human language. It enables machines to understand, interpret, and generate human-like text, making it essential for applications like virtual assistants and language translation. - General AI (Strong AI):
General AI, or Strong AI, is a theoretical concept in Artificial Intelligence that envisions machines possessing the ability to perform any intellectual task at a level equal to or beyond that of a human being. Unlike Narrow AI, which is designed for specific tasks, General AI would have the capacity to adapt and excel across a broad spectrum of activities, demonstrating true cognitive flexibility and understanding. - Generative AI (Gen AI): Generative Artificial Intelligence refers to a subset of artificial intelligence that involves systems capable of producing new content, data, or artifacts. Unlike traditional AI models that focus on recognition and classification tasks, generative AI aims to create novel outputs, often in the form of images, text, or other types of data. This approach is frequently associated with techniques such as Generative Adversarial Networks (GANs) and variational auto-encoders, which enable machines to generate realistic and creative content by learning patterns and structures from large datasets. Generative AI holds promise in various fields, including art creation, content generation, and even the synthesis of realistic, but entirely artificial, data.
- Predictive AI: Predictive Artificial Intelligence refers to the use of advanced algorithms and models to analyze historical data, identify patterns, and make predictions about future events or outcomes. This subset of artificial intelligence is particularly focused on forecasting and anticipatory decision-making. Predictive AI systems leverage machine learning techniques to learn from past data, discern trends, and develop models that can be used to predict future trends, behaviors, or events. Common applications of predictive AI include sales forecasting, risk management, demand planning, and personalized recommendations. By understanding patterns in data, predictive AI empowers businesses and organizations to make informed decisions and proactively respond to potential future scenarios.